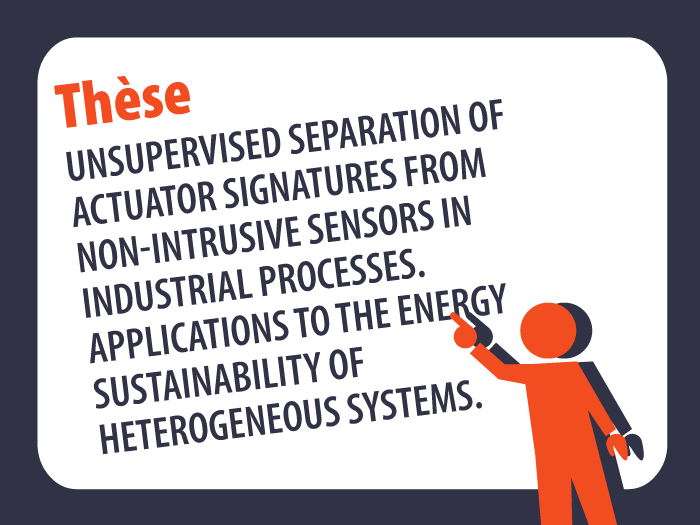
On Friday, December 15, 2023, at 2:30 pm, Mr. Romain Delabeye will defend his thesis entitled Unsupervised separation of actuator signatures from non-intrusive sensors in industrial processes. Applications to the energy sustainability of heterogeneous systems. The work was directed by Jean-Luc Dion and Olivia Penas, and supervised by Martin Ghienne.
Abstract
The manufacturing industry is responsible for a large part of the world’s energy consumption. Many factory pilots seek to reduce their energy-related footprint, while maintaining or improving their productivity. This entails several industrial challenges and scientific issues. (i) How to retrieve a production process from non-intrusive sensor data in order to assess a sub-system’s energy performance in a scalable fashion? (ii) To have a better understanding of a system’s energy dynamics: can energy transfers, local losses and storage effects, be estimated and automatically modeled in a production system without prior knowledge? (iii) To what extent is a scientific approach applicable to heterogeneous industrial use cases? This also raises the issue of an early verification and validation methodology, enabling enhancements to be made prior to any implementation, as system availability and cost of experiments are often prohibitive. Due to the large number of machines in a factory, these research questions are subject to the constraint of non-intrusive sensors and unsupervised methods, so that the solutions sought can be extended to entire production lines and plants. Following a scientific methodology, this thesis addresses these issues with a series of methods aiming at delivering better energy monitoring capabilities for the manufacturing industry. The solution is sought as a stand-alone system, facilitating the adoption of these methods in industrial environments. A systems engineering approach is used to specify and position the subject in the context of a large collaborative project.
The objective of this thesis is threefold. To begin with, the production process is recovered from sensor data as a sequence of operations involving actuators. Mixed signatures are separated — using dictionary learning techniques for stationary signals and harmonic component tracking for non-stationary signals —, and activation statuses are retrieved in place of the actuators’ signals. Actuator signal separation and prediction are therefore entrusted to a model synthesis technique capable of integrating physical constraints. Towards a second objective, a multiphysics model synthesis method based on bond graphs is proposed to recover a system’s energy transfers, together with an extensive literature review on model synthesis approaches. Addressed with ontology-based methods, the third objective aims to assess the applicability of scientific approaches to specific use cases, and enable design improvements as part of a verification and validation process.
Scientifically, this research unveiled several fundamental issues in the field of data-driven modeling. The first issue concerns semi-binary matrix factorization techniques in the complex plane for the recovery of activation sequences. A detailed study shows that the phase induces multiple minimizers of which only one is significant, and a solution is proposed to reach it through means of regularization. A second issue concerns the sequential Monte Carlo probability hypothesis density filter. Particularly well-suited to multi-target tracking in presence of false alarms and nonlinearities, this filter nevertheless presents degeneracies. A new degeneracy phenomenon in particle filters caused by missed detections is identified in experiments, and tackled with a feature-aided approach. At last, energy separation into dissipated and stored energy is addressed with preliminary approaches, but is shown to be ill-posed in the general case.
Along with this thesis, laboratory and synthetic experiments have been designed. The CAFFEINE dataset was produced as a collection of non-intrusive sensor measurements on a coffee machine. The extent to which this use case is representative of industrial production systems is carefully studied. An open source library is also proposed for nonlinear bond graph modeling and simulation.
Key words
# Blind source separation
# Ontologies
# Unsupervised learning
# Multi-physics systems
# Energy sustainability
# Verification and validation